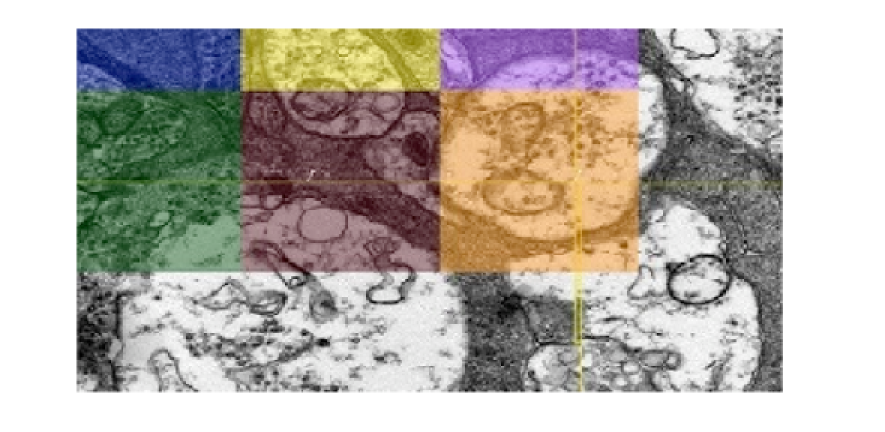
Background
The multiscale/multiphysics nature of materials gives rise to extremely challenging and unique mathematical and algorithmic problems currently prohibiting uncertainty quantification and predictive materials modelling. For example, information loss during coarse graining; high-dimensionality and scalability of stochastic multiscale simulations and UQ; learning in models with a high dimensional parameter space; rare events; transferability of potentials in electronic structure, atomistic and molecular simulations. Materials of interest include, but are not limited to, metallic alloys, ceramics, polymers and composites, semiconductors, biological and geological media and meta-materials. The ability to predict the properties of these materials with confidence is paramount to a number of sectors. Currently there is a huge gap between state-of-the-art mathematical methods and the analysis tools routinely used by the materials community.
Objectives
The primary aim of the workshop was the identification and integration of synergistic methodologies from different research fields (including materials physics and chemistry, uncertainty quantification and machine learning) towards predictive materials modelling. Considering the significant advances in materials characterisation techniques, the workshop emphasised:
- Mathematical and statistical developments for a data-driven approach to predictive materials modelling;
- Accelerated design of materials and processes in the presence of inherent, structure, process, model and epistemic (e.g. limited data) uncertainties.
Scientific Focus
The workshop brought together perspectives from scientists in computational materials physics/chemistry, uncertainty quantification, and machine learning communities. The focus of the workshop was on identifying needed advances on fundamental mathematical, statistical and machine learning approaches to impact data-driven predictive multiscale materials modelling and design. Particular topics of interest included:
- Probabilistic hierarchical and multiscale models of material structure: learning from Bayesian advances in image processing;
- Scalable UQ for multiscale materials models, Probabilistic Graphical Models, Deep Networks, non-parametric learning/inference algorithms;
- Network theory as an approach to modelling and interpreting materials phenomena, modelling of rare events;
- Constitutive model and pseudo-potential errors and transferability, errors and UQ in DFT;
- Stochastic models in molecular systems, accelerated simulation methods for high-dimensional stochastic models;
- Coarse-graining, variational approaches and model selection challenges, non-equilibrium systems;
- Modelling of defects in random environments;
- Data-driven surrogate models for materials modelling and design (modelling of epistemic uncertainties)
- The Materials Genome, structure/property descriptors, exploring high-dimensional process/property/structure relations.
- Building surrogate models for the analysis and design of particular QoI (quantity of interest) using the most informative multiscale simulations (active learning).
Industrial Relevance
Many sectors stand to benefit from mathematical innovations which lead to rapid materials development and deployment. This workshop provided opportunities to begin bridging existing knowledge gaps by identifying and addressing key fundamental problems towards predictive materials modelling and design. For example, the timeliness and cost-effectiveness of materials engineering processes for new designs and design changes can be radically improved by replacing today's experimentally-based materials qualification process with modelling and probabilistically-based materials engineering.
The methods discussed in the workshop have significant potential to reduce the cost, time, and risk of material system validation for advanced materials, assessment of new design configurations at the component level, and for assessing risk and capability at the system level. In addition, the workshop explored the benefits of data-driven simulation of industrial material systems. This is critical for materials design/development and prognosis of failure to many industries (aerospace, energy research, bio-pharmaceutical) where the ability to collect structure and property data at different resolutions and scales has become widely accessible, but still with limited integration of data with process modelling and design.
This event brought together perspectives from national and international leading scientists in computational materials physics and chemistry, uncertainty quantification (UQ) and computational statistics/machine learning communities. It was also of interest to industrial participants from a range of sectors including:
- Aerospace;
- Pharmaceuticals;
- Electronics;
- Automotive;
- Energy (including environmental remediation).
Dissemination
In addition to the presentation slides, most lectures and discussions were video-recorded and are made publicly available through the Programme tab above.
Following the event, the Warwick Centre for Predictive Modelling compiled a comprehensive report that can be read on the KTN website.
Furthermore, a special issue of the Journal of Computational Physics is planned that will include review articles and specific works demonstrating the integration of methodologies from Computational Mathematics, Uncertainty Quantification, Machine Learning and Multiscale modelling towards predictive materials modelling.
Registration, Venue and Accommodation
The workshop took place at the Isaac Newton Institute for Mathematical Sciences in Cambridge. Please see the Isaac Newton Institute website for further information about the venue. Registration was open to researchers and industry representatives from relevant/related fields.
There were opportunities for networking and investigating of potential collaborations and the event included a drinks reception and college dinner.
Feedback
If you attended the event, but did not complete a feedback form, it would be helpful if you could do so.
Further Information
This activity was a partnership of the Warwick Centre for Predictive Modelling, the Isaac Newton Institute for Mathematical Sciences (INI), the Turing Gateway to Mathematics and the Knowledge Transfer Network.
The academic organisers included Prof. Nicholas Zabaras (University of Warwick) and Markos Katsoulakis (University of Massachusetts at Amherst). For further details please contact Jane Leeks at the TGM.